The Clinical Machine Learning Lab is interested in developing novel machine learning and artificial intelligence methods and using these techniques to advance health care. Our current on-going research projects include:
- MR Fingerprinting in Epilepsy
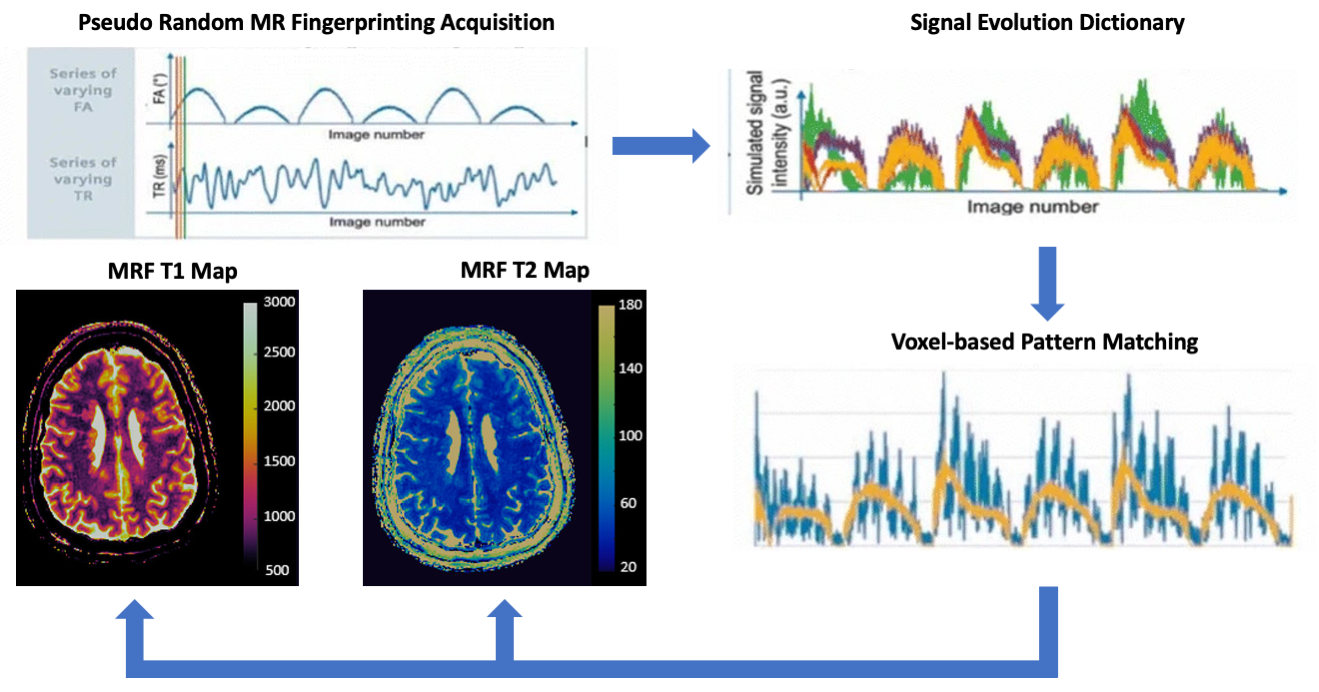
Epilepsy affects 65 million people worldwide; approximately 30% of them do not respond to medications but can be cured by surgery. Conventional MRI can be limited in detecting subtle epileptic lesions or identifying active/epileptic lesions among widespread, multifocal lesions. We use the novel Magnetic resonance fingerprinting (MRF) technique to generate high-resolution quantitative tissue property maps (T1 and T2 maps). We are developing MRF-based radiomic machine learning models to actually predict epilepsy outcomes.
Funding: NIH NINDS
- MR Fingerprinting in Brain Tumors
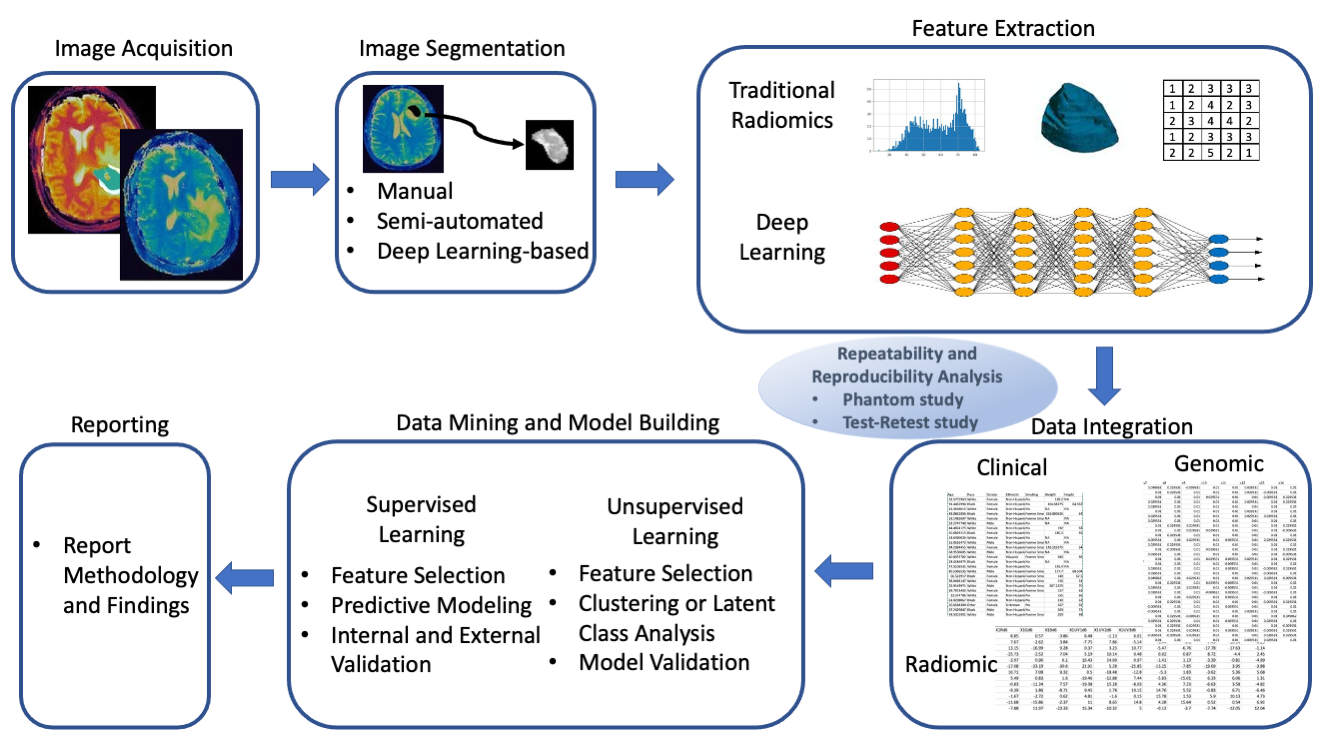
This project focuses on the development and clinical translation of an MR Fingerprinting based quantitative imaging and analysis platform, with a specific application for glioblastoma infiltration prediction for targeted surgery or radiation therapy.
Funding: NIH NCI
- RWD and RWE Research in COVID-19
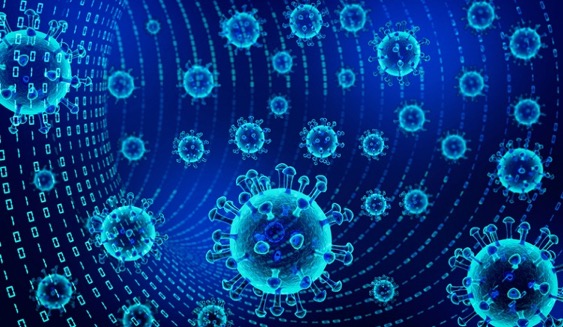
COVID-19 has created an unprecedented challenge to public health and security, resulting in an urgent need for the rapid development and application of innovative methods. Real-world data (RWD) and real-world evidence (RWE) offer the ability to better understand (and use) COVID-19 therapeutics and diagnostics. We are conducting multiple research projects on COVID-19 outpatient treatments, COVID ICU treatments, and long COVID conditions using Cleveland Clinic real-world electronic health record data.
- Clinical NLP
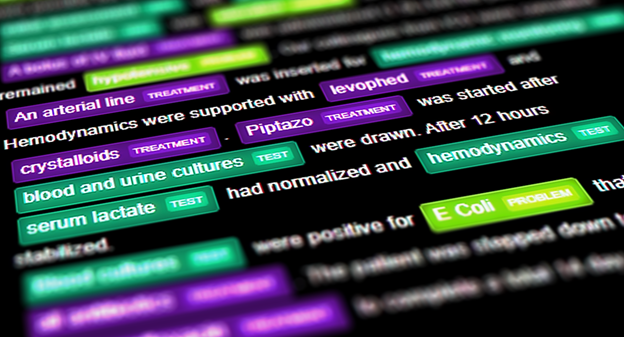
Natural language processing (NLP) can extract coded data from clinical text, making historical clinical unstructed information available for analytics and modeling. With the recent algorithm improvements, NLP is more powerful and accessible than ever before in clinical informatics. Our group are developing clinical NLP pipelines using modern transfer learning and semi supervised learning techiques.